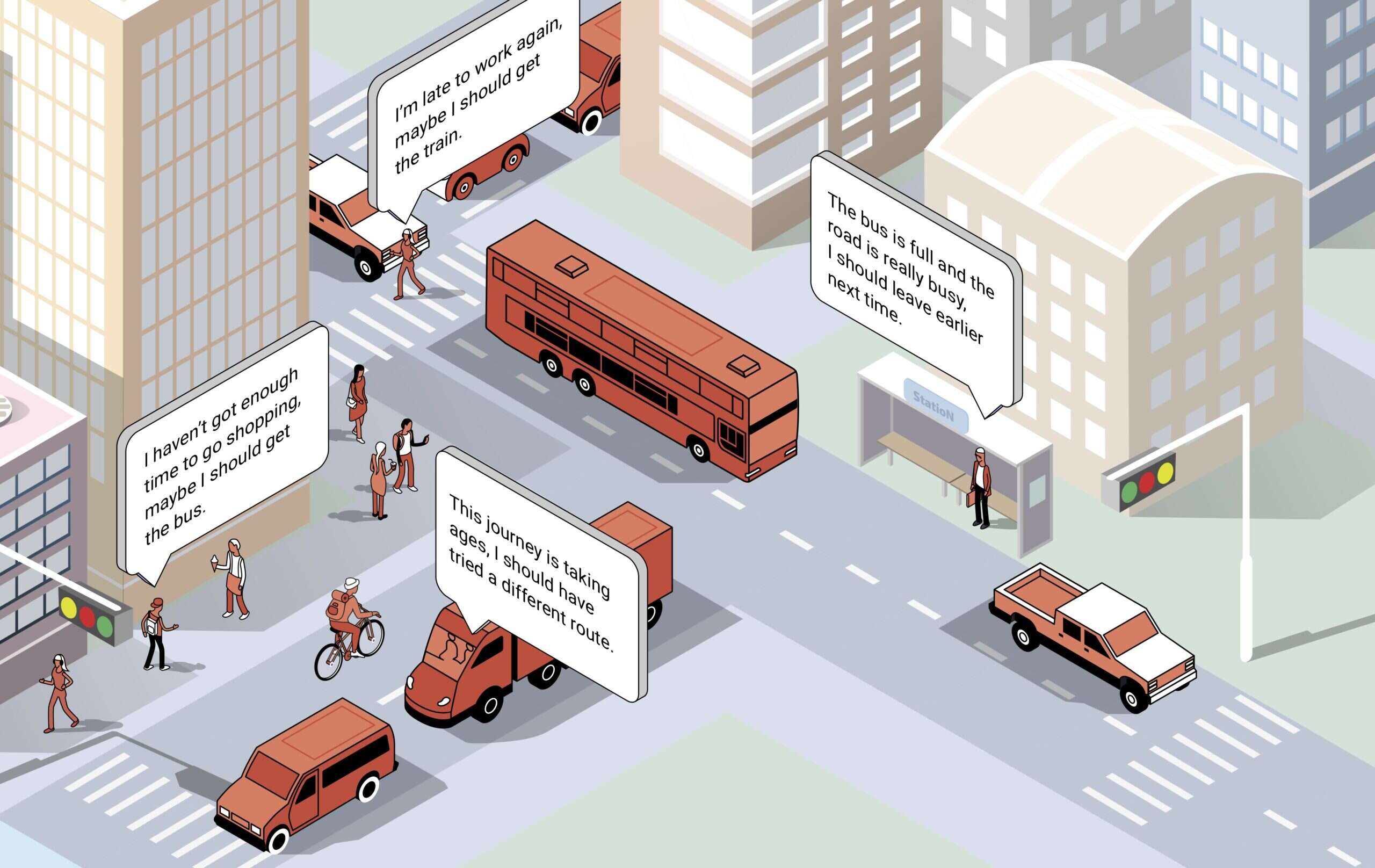

Assumptions regarding how people move and behave have grown increasingly inaccurate in the past decade, but it took Covid-19 for people to truly pay attention to these flaws. Yet these assumptions continue to form the foundation of global transport systems.
Complexity and variability have become the new normal as a result of the pandemic. But current systems for predicting passenger travel fail to allow for that. Take for example the day of a working parent. Existing outdated modelling assumes they drop off their child at the nursery and then head to the office. But in reality, the typical nine-to-five has disappeared, and their day might involve a nursery drop-off, followed by a trip to the grocery store before an afternoon of telecommuting. How do we plan transit around that trajectory?
Uncertainty triggered by Covid-19 has created a magnitude of challenges. Fewer trips are being taken overall, and despite lockdowns, traffic is near pre-coronavirus levels. Fewer people are taking trains and buses, which points to a potential mode shift towards cars. In fact, according to data from the Google Mobility Report, public transport use in London was 29% of pre-Covid 19 levels as of mid-December 2020. In the US, the picture is even worse, with ridership down 62% from pre-pandemic levels as of the third quarter of 2020.
Covid-19 is a wake-up call for the industry… only by introducing new technologies and processes will we be able to create the transport system we want and need in these fundamentally unpredictable times.
As the vaccine programme gains momentum and restrictions ease, we face unprecedented levels of uncertainty, with little understanding of future demand. But ambiguity triggered by the pandemic is just one of the industry’s many challenges. Emerging technologies and transport modes, such as electric vehicles and electric scooters, as well as the need to decarbonise to meet net-zero commitments, and address social inequality, are all contributing to an unprecedented strain on the network.
Existing travel prediction systems are not designed for the level of interdependent changes we face in the immediate future. They are based on a narrow set of aggregated data to predict human behaviour that has been collected over the past 50 years. While this can provide high-level estimates for transport service performance and anticipate changes and pinch points – such as disruption to morning and evening peaks or high-level estimates for headway on major roads during peak hours – it does not help us understand changes in conditions such as mode shift and emerging travel patterns like e-mobility.
Agent-based modelling
In preparation for the post-Covid world, transport leaders need to invest in a better and more efficient forecasting system – an agent-based model (ABM). This technology allows planners to consider each traveller as an individual with unique characteristics and goals. It uses data to simulate what happens as groups of these unique individuals travel through systems, and understanding individual behaviours is vastly beneficial.
Implementation of an ABM would create better transit service and reliability. It could help to ensure seamless integration between traditional modes of transport and newer ones. It would also be better for the environment: if we are able to forecast new travel patterns, we can support land-use plans to minimise trips in personal cars. The modelling also would give us answers to complex questions concerning the impact of implemented changes, which in turn could help create a more equitable transport system.
Consider the example of a new road tolling system. Current prediction systems will fail to anticipate consequences on social welfare. ABMs would help us explore any unintended effects of the new pricing system on certain communities or groups of people, like blue-collar workers, who may not have access to public transport where they live or the option to work from home to avoid additional charges.
Countries across the world have realised the potential of ABMs
Our organisation, Arup, is already working with transport authorities globally using ABMs. We are helping the New Zealand Ministry of Transport build a national simulation capability that models 10% of the country’s population. This project explores how an agent-based model could be used over the next five to 50 years. The plan is to assess policies like road tolling against net-zero emissions targets and inclusive-access targets. An ABM would allow the ministry to fully understand who is impacted by various policy scenarios. We are also working with Transport Infrastructure Ireland (TII) using agent-based modelling to develop a plan for a sustainable funding model that is able to respond to the everyday network demands while providing support for more sustainable and equitable policy ambitions. This work will result in greater foresight for TII into the challenges and opportunities that the national road network faces.
We have also partnered with Transport for London (TfL) to assess what an agent-based model for that city would require. To do so, we built a behaviour model representing one million unique people. Each person made 750 decisions in a day. Taken in aggregate, we can simulate and measure congestion, public transit demand and where people spend time (home, work, leisure, for example). This type of modelling could enable TfL to simulate the impacts of yet-to-be-fully-implemented technology changes, which we do not have any precedent for, like connected autonomous vehicles. The research demonstrates the viability of an agent-based approach, even in large and complex transport systems like London’s.
Rebuilding transit after Covid
ABMs could also be used to understand a range of Covid-19 recovery scenarios, estimating their short and medium-term impacts on public transport, traffic and demand for cycling infrastructure.
Covid-19 is a wake-up call for the industry to invest in new modelling systems to create more efficient, community-centric and sustainable transport networks. The current method of using outdated assumptions stands in the way. Only by introducing new technologies and processes will we be able to create the transport system we want and need in these fundamentally unpredictable times.